The Hasson lab aims to investigate the underlying neural basis of natural language acquisition and processing as it unfolds in the real world.
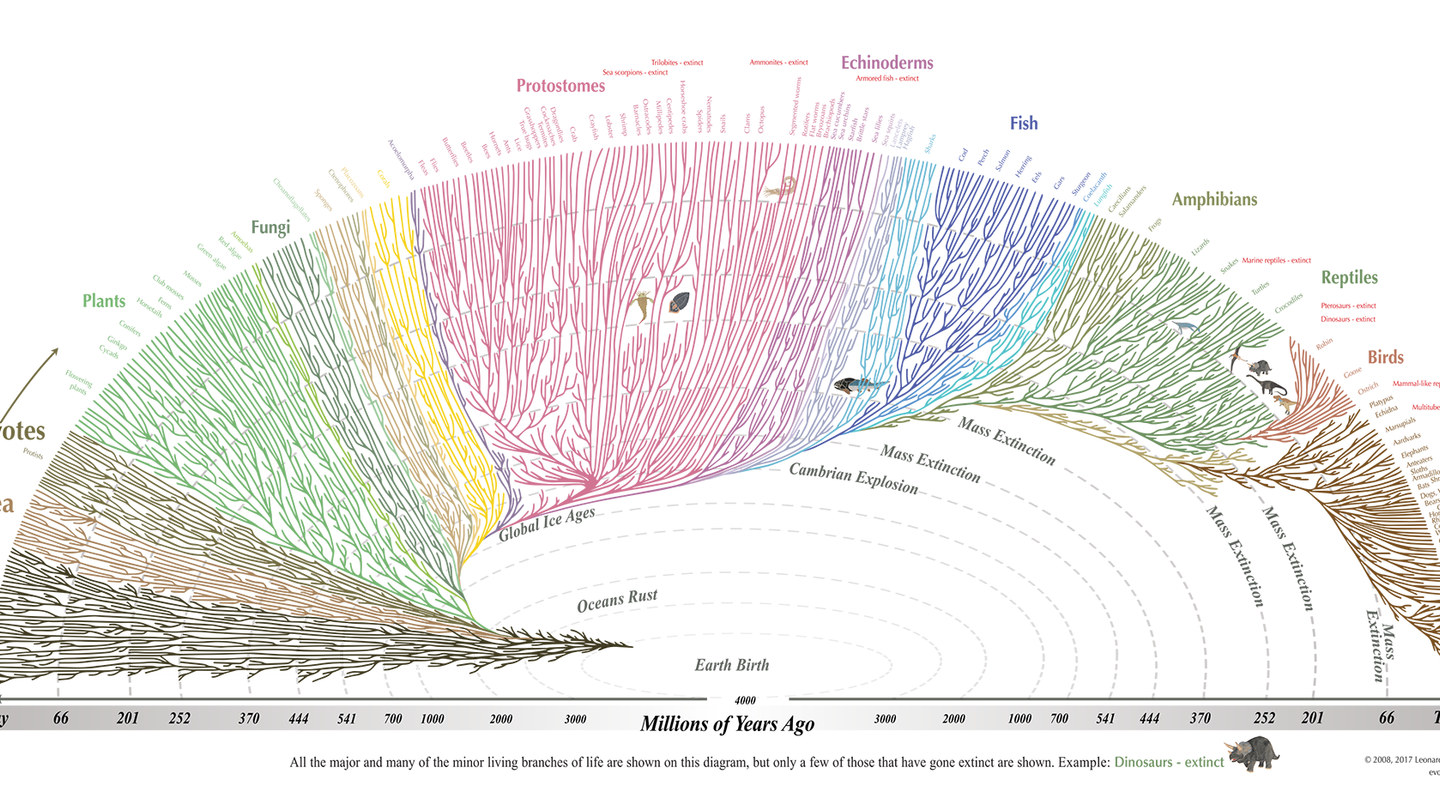
Neuroscience in the real-world
Cognitive neuroscientists employ clever experimental manipulations to explain the relationship between the brain, behavior, and the environment. We hope that the models we derive from tightly-controlled experimental manipulations will provide some traction in real-world contexts. This belief relies on the assumption that the human brain implements a set of rules that capture the underlying principles by which the world works. We assume that these rules, like in classical physics, are relatively simple and interpretable and, once discovered, will extrapolate to the richness of human behavior. To discover these rules, we filter out as many seemingly irrelevant variables (considered “confounds ”or “noise ”) in hopes of isolating the few essential latent variables ( “signals ”) that dictate brain-behavior relationships. But to what extent do our experiments uncover simple rules that can be generalized to human behavior outside the laboratory?
Our studies of neural responses to natural stimuli (such as audio-visual narratives) led us to realize that models developed under particular experimental manipulations in the lab fail to capture variance in real-life contexts (see, for example, our work on process memory). The Hasson Lab aims to develop new theoretical frameworks and computational tools to model the neural basis of cognition as it materializes in the real world.
Further Reading
Deep learning as cognitive models for the real world
Deep learning and artificial neural networks provide an alternative computational framework to model cognition in natural contexts. In contrast to the simplified and interpretable hypotheses we test in the lab, deep neural networks learn to perform complex cognitive tasks in real-life contexts without simplifying the input or relying on predefined psychological constructs. Counterintuitively, like evolutionary processes, over-parameterized deep neural models are parsimonious and straightforward, providing a versatile, robust solution for learning diverse cognitive functions in natural contexts.
Further Reading
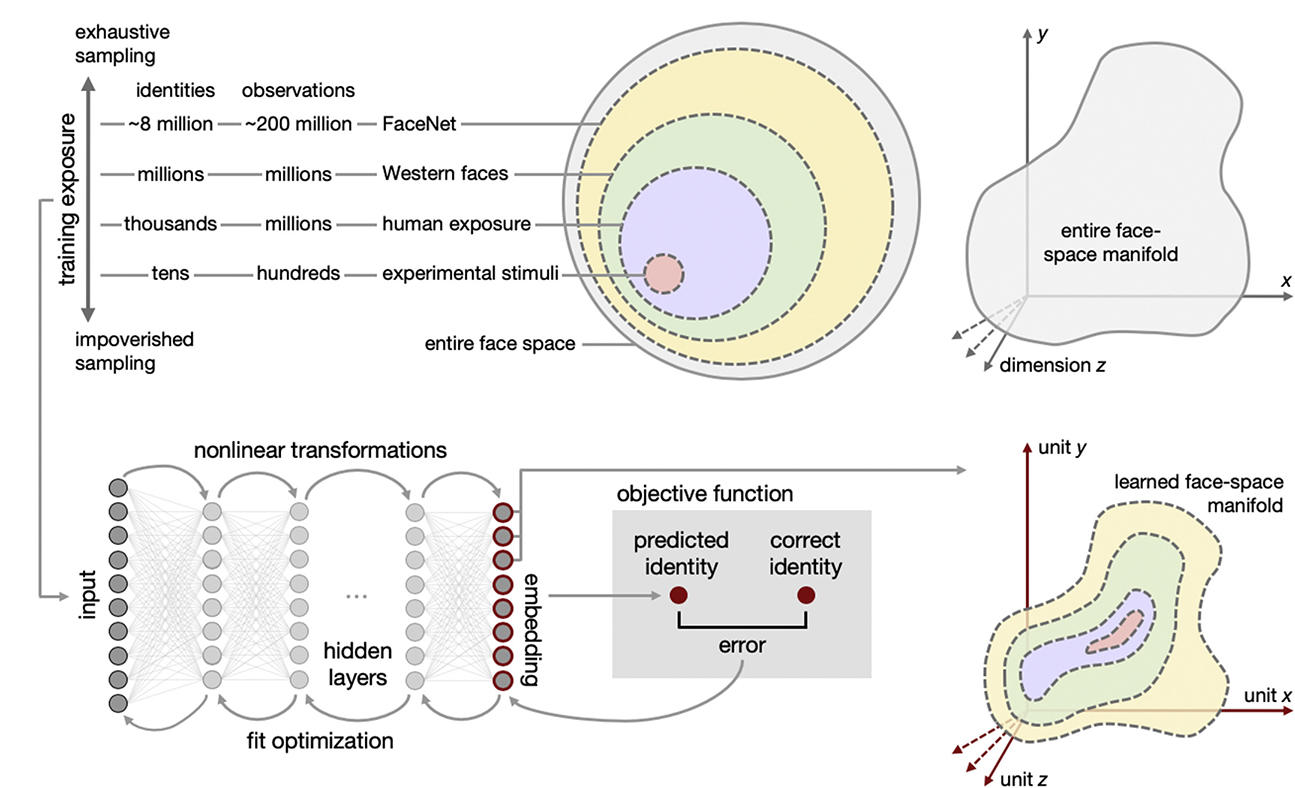
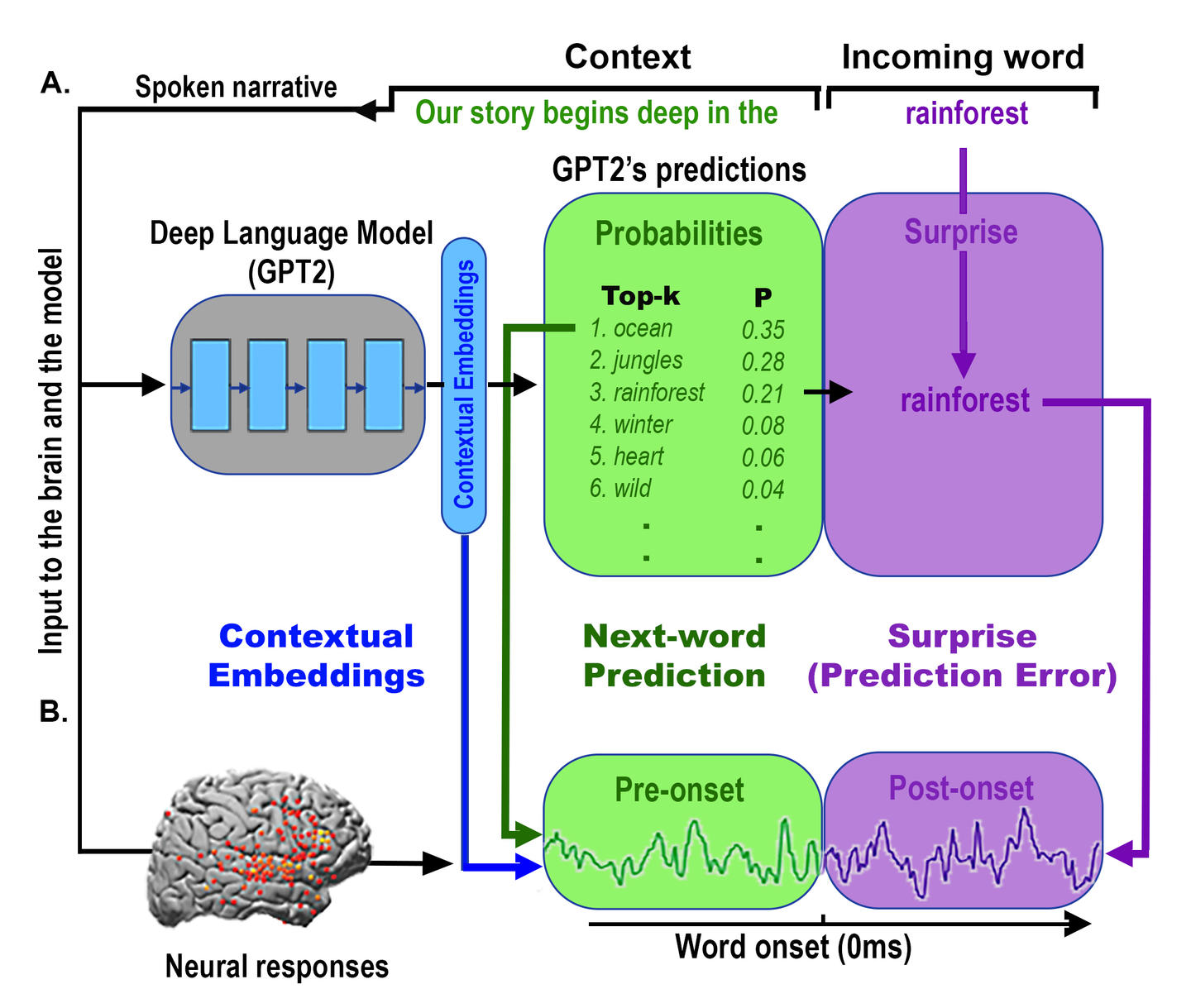
The neural basis of daily conversations
Natural language processing - that is, the way we use language to communicate our thoughts in daily life - is a prime example of the tension between our interpretable theories and the messiness of the real world. The lab aims to model brain responses as people engage in open-ended conversations. Our data-set consists of ECoG data (intracranial EEG) collected continuously from the brains of epileptic patients as they were engaged in open-ended, free conversations with their doctors, friends, and family members during a week-long stay at the hospital.
Traditionally, the investigation of the neural basis of language relied on psycholinguistic models, which use explicit symbolic representations of parts of speech (like nouns, verbs, adjectives, adverbs, and determiners) combined with rule-based logical operations embedded in hierarchical tree structures. Classical symbolic-based linguistic models, however, fail to capture the multitude of linguistic variations in the real world. Advances in deep language models provide a new theoretical framework for modeling natural language processing in the human brain. We currently explore whether we can use deep language models as a cognitive model to explain natural language processing in the human brain. In particular, we are searching for shared computational principles and inherent differences in how the brain and deep neural networks process natural language. Overall, our findings suggest that deep language models provide a new and biologically feasible computational framework for studying the neural basis of language.
Further Reading
The First 1,000 Days Project
All babies are born helpless, equipped with minimal cognitive-motor skills, and must rely on their caregivers to provide for their needs. However, within a few years, they walk, climb, talk, and learn to reason about the world, albeit with enormous variation from child to child. What mechanisms allow the child to develop autonomous motor and cognitive abilities over its first 1000 days? One of the biggest impediments to modeling development change across time is the lack of dense measurements of children’s lives as they grow. Developmental science is currently limited by a broad reliance on sparse and narrow behavior sampling for a few minutes, mostly in highly controlled experimental settings. A comprehensive, longitudinal dataset is needed that quantifies the richness of sensory input and the closed-loop interaction between the children's behavior and their physical and social environment.
Princeton’s 1kD project aims to collect dense child-centric data for modeling child development in the real world. In collaboration with Casey Lew-William and Princeton’s Baby Lab, we have built a large-scale automatic pipeline to monitor the daily lives of 15 babies for 12 hours a day throughout their first 1,000 days of life. We record the baby's life in each house using 12 cameras and microphones. Using modern machine learning models, we automatically detect and process minutes in which the baby interacts with its environment. Quantifying children's development over time lays the groundwork for building novel computational models of how humans learn a language and develop cognition by interacting with their social environment during the first 1,000 days of life.
Princeton’s First 1,000 Days Project is part of Wellcome Leap’s multidisciplinary research initiative.
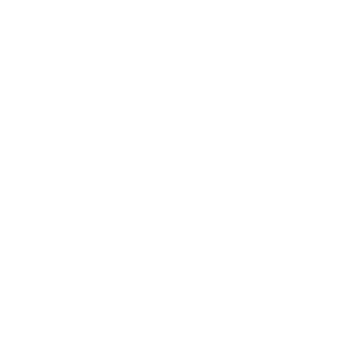
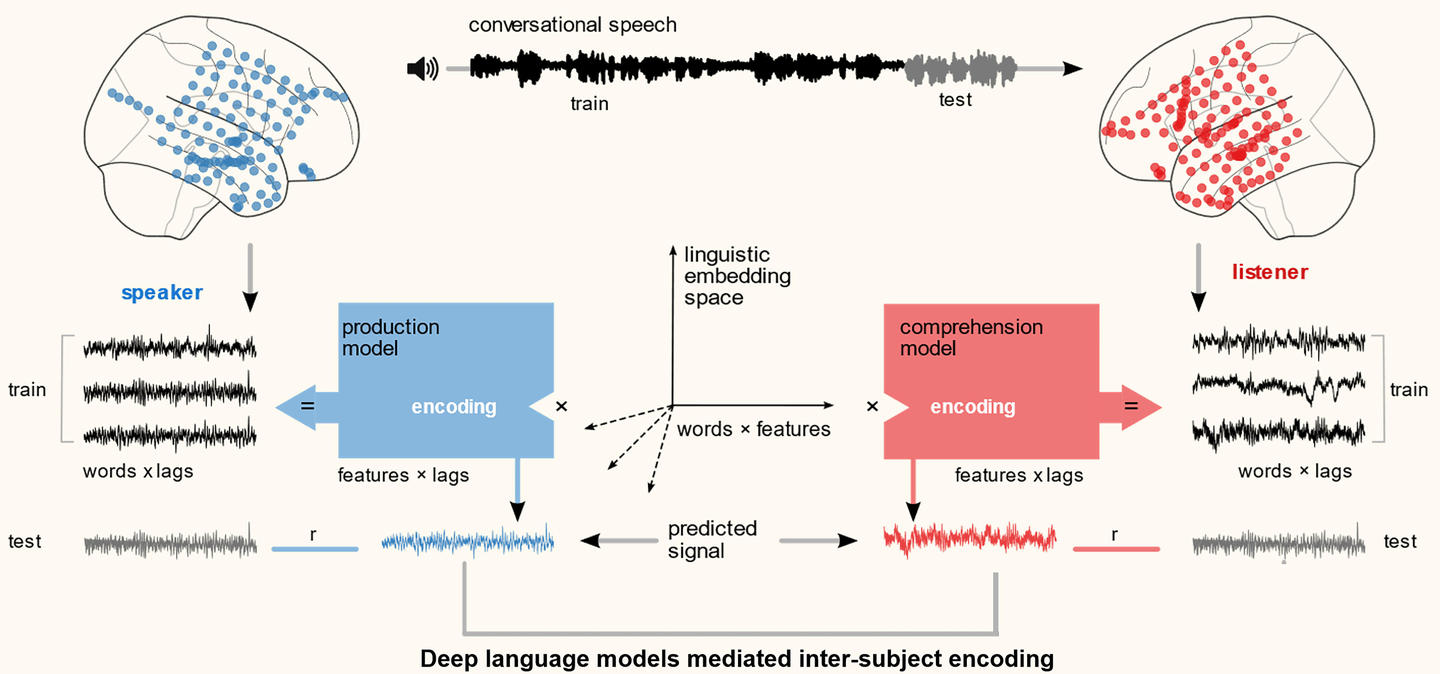
Face to Face, Brain to Brain: Exploring the Mechanisms of Dyadic Social Interactions
Cognition materializes in an interpersonal space. Little is known about the neural substrates that underlie our ability to communicate with other brains in naturalistic settings. We are developing novel methodological and analytical tools for characterizing neural responses between speaker and listener - during the production and comprehension - of complex real-life speech. We use fMRI and ECoG hyperscanning methods to measure the neural coupling during natural communication. We study the speaker-listener neural coupling during storytelling, open conversations, and teacher-student structured interaction. Our studies start to uncover the shared underlying neural mechanisms at the basis of the ability to transfer our thoughts, memories, and emotions to others.
Further Reading
Topographic mapping of a hierarchy of temporal receptive windows
In everyday life, memories of the past are constantly integrated with presently incoming information. Consider, for example, how present and prior information converge when listening to speech: each phoneme achieves its meaning in the context of a word, each word in a sentence, and each sentence in a discourse. Comprehension of each word, sentence, or idea also relies on knowledge accumulated in the listener’s brain over many years. However, there is little research on the neural processes that allow the brain to gather information over time. In collaboration with Ken Norman, the long-term goal of our laboratory is to understand how the brain uses information accumulated and integrates information across multiple timescales, ranging from milliseconds to days, to make sense of moment-to-moment incoming information.
Further Reading
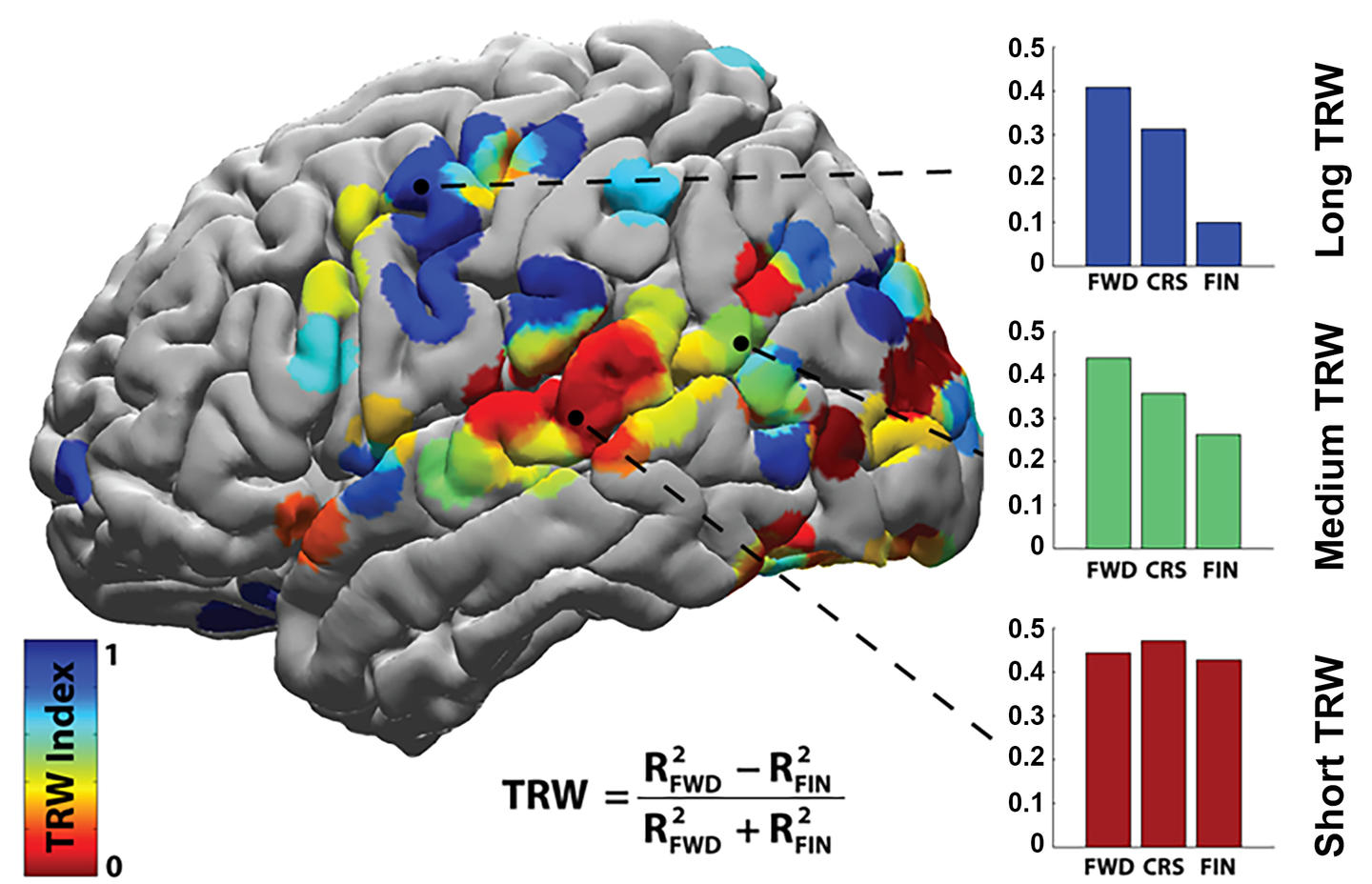